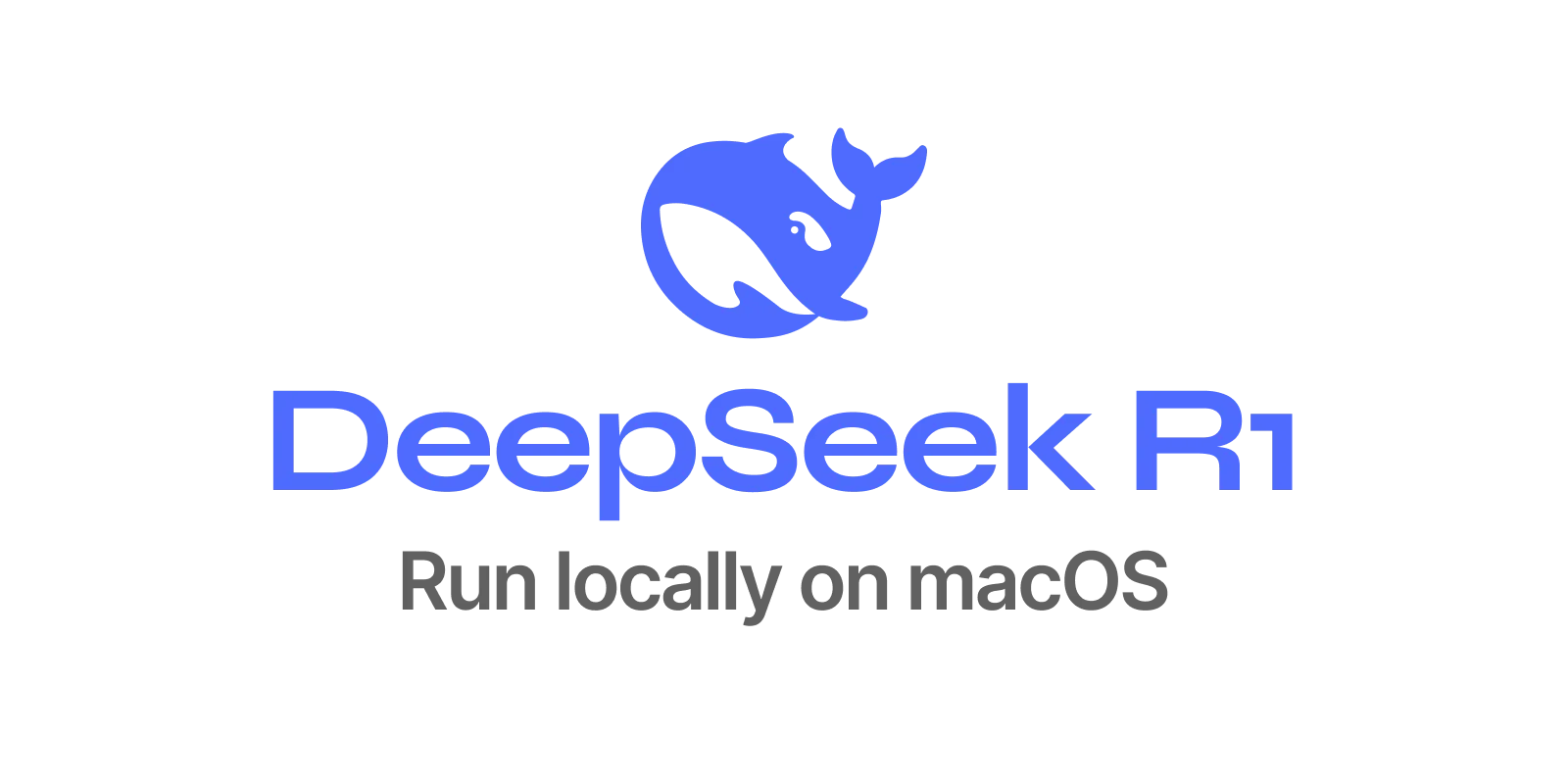
Run DeepSeek R1 locally on macOS
What is DeepSeek R1?
DeepSeek R1 is a first-generation reasoning model developed by DeepSeek AI that excels at complex reasoning tasks with a similar performance to OpenAI-o1 model. It demonstrates remarkable capabilities in mathematics, coding, and problem-solving, making it a powerful tool for both researchers and developers.
Benefits of Running Locally
Running DeepSeek R1 locally on your Mac offers several advantages:
- Privacy: Your data stays on your device and doesn't go through external servers
- Offline Usage: No internet connection required once models are downloaded
- Cost-effective: No API costs or usage limitations
- Low Latency: Direct access without network delays
- Customization: Full control over model parameters and settings
For macOS users, options to run LLMs like DeepSeek R1 include using specialized platforms such as Ollama or LM Studio, which make it easy to download and run models without complex setups.
Models and Hardware Requirements
Here's a breakdown of popular DeepSeek R1 models available on Ollama, along with their approximate sizes and hardware recommendations:
Model | Parameters | Size | VRAM (Approx.) | Recommended Mac |
---|---|---|---|---|
deepseek-r1:1.5b | 1.5B | 1.1 GB | ~2 GB | M2/M3 MacBook Air (8GB RAM+) |
deepseek-r1:7b | 7B | 4.7 GB | ~5 GB | M2/M3/M4 MacBook Pro (16GB RAM+) |
deepseek-r1:8b | 8B | 4.9 GB | ~6 GB | M2/M3/M4 MacBook Pro (16GB RAM+) |
deepseek-r1:14b | 14B | 9.0 GB | ~10 GB | M2/M3/M4 Pro MacBook Pro (32GB RAM+) |
deepseek-r1:32b | 32B | 20 GB | ~22 GB | M2 Max/Ultra Mac Studio |
deepseek-r1:70b | 70B | 43 GB | ~45 GB | M2 Ultra Mac Studio |
deepseek-r1:1.5b-qwen-distill-q4_K_M | 1.5B | 1.1 GB | ~2 GB | M2/M3 MacBook Air (8GB RAM+) |
deepseek-r1:7b-qwen-distill-q4_K_M | 7B | 4.7 GB | ~5 GB | M2/M3/M4 MacBook Pro (16GB RAM+) |
deepseek-r1:8b-llama-distill-q4_K_M | 8B | 4.9 GB | ~6 GB | M2/M3/M4 MacBook Pro (16GB RAM+) |
deepseek-r1:14b-qwen-distill-q4_K_M | 14B | 9.0 GB | ~10 GB | M2/M3/M4 Pro MacBook Pro (32GB RAM+) |
deepseek-r1:32b-qwen-distill-q4_K_M | 32B | 20 GB | ~22 GB | M2 Max/Ultra Mac Studio |
deepseek-r1:70b-llama-distill-q4_K_M | 70B | 43 GB | ~45 GB | M2 Ultra Mac Studio |
Note: VRAM (Video RAM) usage can vary based on the model, task, and quantization. The above is an approximation.
Models ending in q4_K_M
are quantized for lower resource usage.
Step-by-Step Guide to Running DeepSeek R1 locally on macOS using Ollama and Kerlig
-
Install and run Ollama
- Go to ollama.com↗ and download the macOS installer
- Install Ollama on your Mac
- Open Ollama after installation
-
Add DeepSeek R1 model to Kerlig
- Download Kerlig↗ and open it
- Go to Settings → Integrations → Ollama
- In Add Custom Model section:
- Enter a display name (e.g., "DeepSeek R1 7B")
- Enter the model name (e.g.,
deepseek-r1:7b
)
- Click Add
- Toggle the switch to enable and download, wait for download to finish (you can close Settings while downloading continues in background)
-
Run DeepSeek R1
- Open Kerlig
- Enter your prompt - any question you want to ask
- Select newly added DeepSeek R1 7B as model
- Click Run or press Enter
Usage Recommendations
- Choose a model size appropriate for your Mac's specifications
- Start with smaller models first to test performance
- Monitor system resources during initial usage
- Ensure adequate free storage space for model downloads
- Keep Ollama running in the background while using Kerlig
Troubleshooting
- For large models, consider closing other resource-intensive applications
- If a model fails to load, check your available system memory
- Ensure Ollama is running before attempting to use models in Kerlig
- If experiencing issues, try restarting Ollama and Kerlig
Conclusion
Remember that model downloads are persistent - once downloaded, you can use them offline in future sessions.
By following these steps, you can successfully run DeepSeek R1 locally on your Mac.
FAQ: Frequently Asked Questions About DeepSeek R1
Q: Which model size should I choose?
Answer: Consider these recommendations:
- Basic tasks: 1.5B model
- Balanced performance: 7B/8B models
- Maximum capabilities: 14B+ models (if hardware supports it)
- Start with smaller models and scale up if needed
Q: What are the minimum hardware requirements for DeepSeek R1?
Answer: Requirements vary by model size:
- 1.5B model: M2/M3 MacBook Air with 8GB RAM
- 7B/8B models: M2/M3 MacBook Pro with 16GB RAM
- 14B model: M2/M3 Pro MacBook Pro with 32GB RAM
- 32B/70B models: M2 Max/Ultra Mac Studio
Q: How much storage space do the models require?
Answer: Storage requirements per model:
- 1.5B model: ~1.1 GB
- 7B model: ~4.7 GB
- 14B model: ~9.0 GB
- 32B model: ~20 GB
- 70B model: ~43 GB
Q: What is the response speed of different model sizes?
Answer: Speed varies by model and hardware:
- 1.5B model: 1-2 seconds on M2/M3 Macs
- 7B/8B models: 2-4 seconds on 16GB RAM machines
- 14B+ models: 4-8 seconds on higher-end hardware
- Quantized (q4_K_M) versions improve speed by 30-50%
Q: What tasks is DeepSeek R1 best suited for?
Answer: DeepSeek R1 excels at:
- Mathematics and complex problem-solving
- Coding and algorithm challenges
- Scientific reasoning
- Step-by-step explanations
- Analytical thinking tasks
Q: How do different model sizes compare in performance?
Answer: Performance scales with model size:
- 1.5B model: 28.9% AIME accuracy, outperforms GPT-4 on certain math tasks
- 7B model: 55.5% AIME accuracy
- 14B model: 69.7% AIME accuracy, close to larger models
- All sizes demonstrate strong reasoning capabilities due to distillation technology
Q: How does DeepSeek R1 compare to other local LLMs?
Answer: DeepSeek R1 distinguishes itself by:
- Outperforming larger models on math and coding tasks
- Excelling at step-by-step problem solving
- Strong scientific reasoning capabilities
- Efficient resource usage through quantization
- Competitive performance at smaller model sizes
Q: Can DeepSeek R1 run offline?
Answer: Yes, after downloading through Ollama, DeepSeek R1 runs completely offline, providing:
- Complete privacy
- No API costs
- Reliable access
- Lower latency
Q: Can multiple models run simultaneously?
Answer: While possible, it's recommended to run one model at a time for optimal performance, especially on machines with limited RAM.
Q: Does performance differ on Apple Silicon vs Intel Macs?
Answer: Yes, Apple Silicon Macs (M1/M2/M3) offer significantly better performance due to:
- Unified memory architecture
- Optimized ML capabilities
- Specific optimizations for Apple Silicon